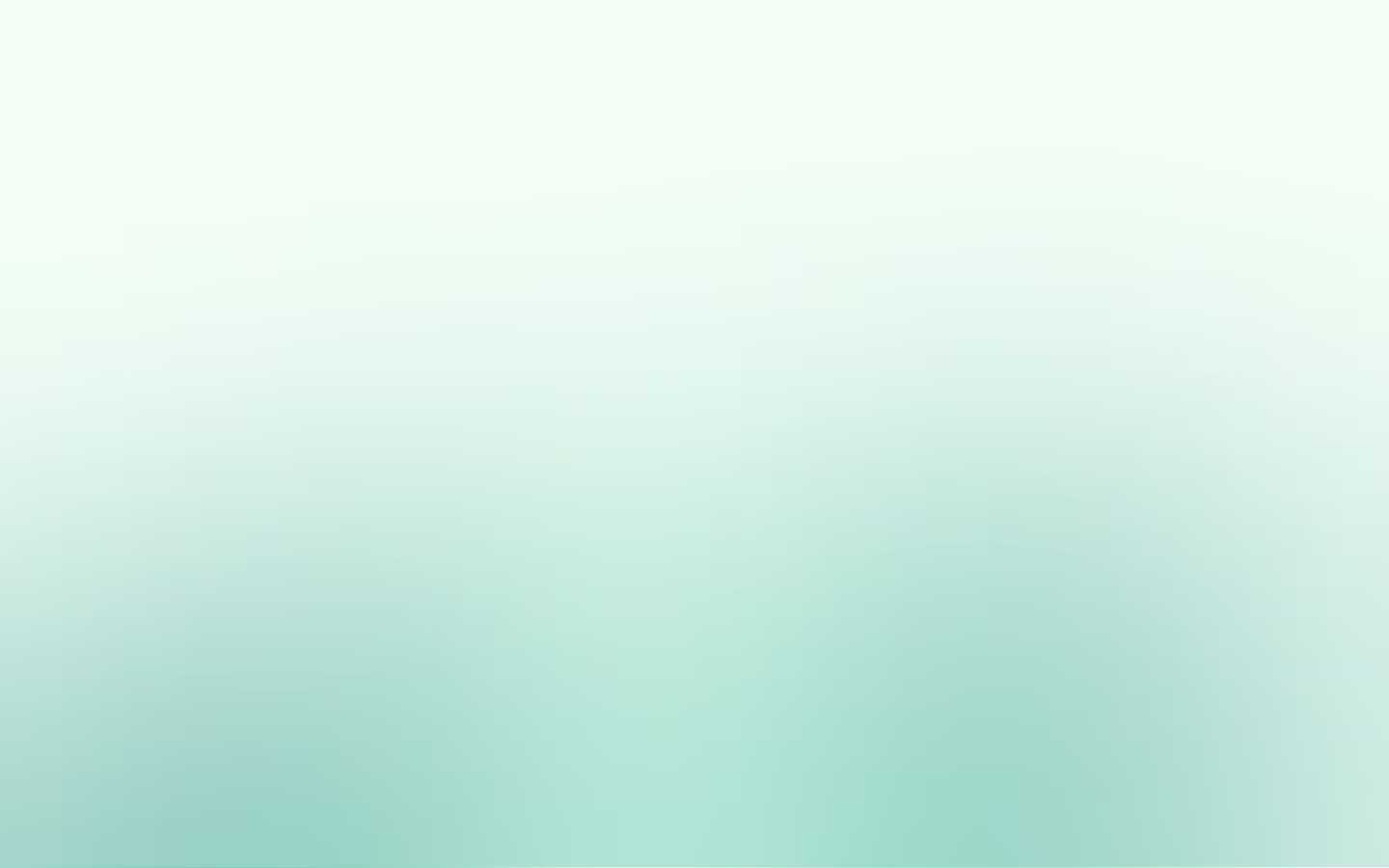
Human expertise and built-in AI Models
The Bytek Prediction Platform uses advanced, customizable artificial intelligence models to enrich customer data, offering useful insights and predictions about customer behavior. Developed by our Data Science team, these models adapt to each company's specific needs and goals.
Schedule a MeetingThe Bytek Prediction Platform uses advanced, customizable artificial intelligence models to enrich customer data, offering useful insights and predictions about customer behavior. Developed by our Data Science team, these models adapt to each company's specific needs and goals.
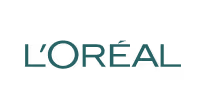
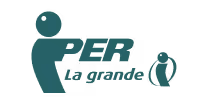
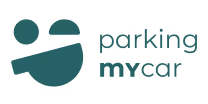



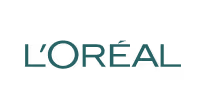
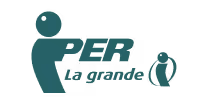
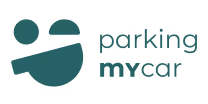



Interests
The model assigns a label indicating a thematic or product interest to each page on the site. Once the labels are assigned, it analyzes how the user navigated through the different interests.

The applied approach is based on embeddings, which transform texts into numerical vectors while retaining their semantic meaning. This transformation makes it possible to compare content and effectively assign thematic or product labels. Then, a proprietary algorithm uses this information to associate interests with users, enabling companies to optimize recommendations, marketing campaigns, and cross-selling and upselling strategies.
Meticulosity
The model assigns interests by analyzing both individual and collective user behavior.
Determinism
The model ensures consistent and replicable results at each run
Cross-sectional Analysis
The model interprets content regardless of its collocation, correctly attributing it to the user.
AI RFM Clustering
The model segments customers by considering their level of engagement with the brand, as measured by recent interaction, frequency of contact and economic value generated.
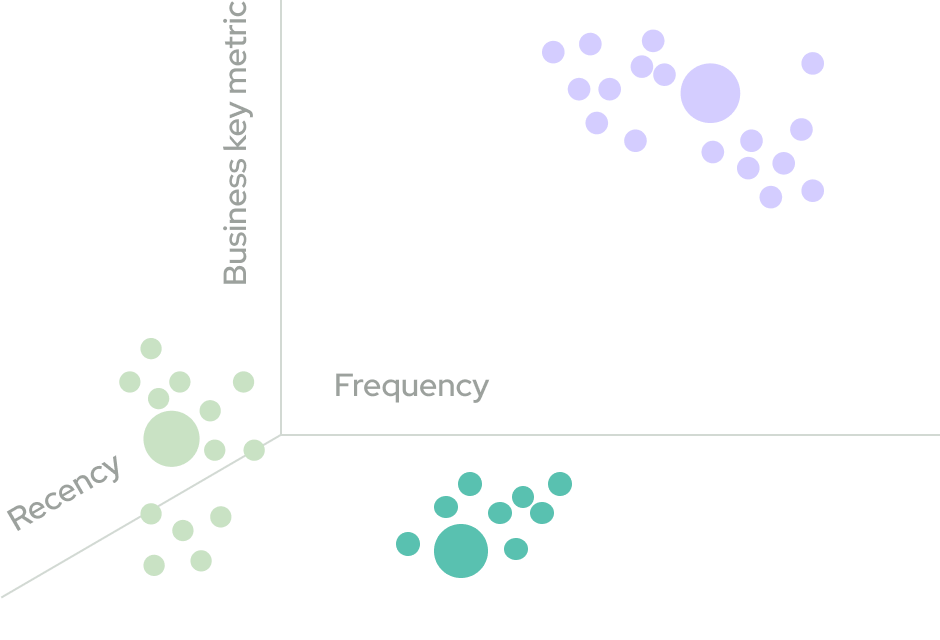
RFM analysis leverages the K-Means algorithm to deliver accurate and efficient segmentation. This approach analyzes multiple customer attributes, handles large data volumes, and dynamically adapts to new inputs, providing precise, scalable, and continuously updated segments. The resulting segments enable companies to focus on their most strategic customers, optimizing targeted campaigns and engagement initiatives.
Customized clusters
The model divides customers into specific categories, defined according to the company's requirements and goals.
Flexibility
The monetary variable is customizable according to business needs, allowing metrics such as profit margin to be analyzed.
Continuous Learning
The model updates with new data, ensuring always current segmentations and rapid response to changes in user behavior.

Ready to talk about AI?
Speak with one of our experts to discover how optimize your strategies with our platform.
Sign Up for a DemoAction Prediction
A predictive model that assigns each lead a score reflecting their probability of taking a specific action, such as making a purchase, subscribing to a newsletter, or scheduling an appointment.

The analysis integrates CRM information with behavioral data, such as pages visited, sessions, channels, events, and downloads. After dimensionality reduction, the most relevant variables are selected for modeling. Next, different approaches are tested, including data-driven, shrinkage and ensemble methods. The process concludes with a validation phase to identify the model best suited to provide representative results for the brand.
Predictive Accuracy
The selection of variables affecting the probability of an action is made according to client characteristics, ensuring accurate results.
Variables Calculated
Key variables for predicting a lead's action are often calculated, such as interests or clusters, identifiable through the platform's other models.
Adaptability
The model is selected considering computational efficiency, speed of execution and, most importantly, a thorough preliminary analysis of the data.
Predictive LTV
The model estimates a customer's lifetime value based on their past behavior and transactional data, helping brands identify the most valuable customers and plan long-term strategies more effectively.

The estimation of predictive LTV is based on three key aspects: the average monetary value per customer, the number of products purchased within a given period and the probability of customer retention in the following months. These values are estimated using a combination of probabilistic distribution methods and classification algorithms.
Sophistication
The model integrates several methodologies, ensuring more accurate and reliale predictive estimation.
Timing Accuracy
Forecast times are calculated very accurately based on the availability of customer data
Rigorous Training and Validation
These steps are conducted with the utmost care to ensure reliable predictions of future customer behavior.