Today we frequently talk about first-party data, but what precisely are they? First-party data can be divided into two main categories:
- Observed data: includes information such as customers’ age, preferred store, date of last purchase, items purchased, and amount spent. This aggregated data can be used to power artificial intelligence, creating personalized user experiences and accurate predictions.
- Modeled data: this data is derived from observed data but is obtained using data mining techniques.For example, it is possible to aggregate all of a user’s interactions with a website to identify their main interests, predict the risk of abandonment, or determine which cluster they belong to, whether they are a top or loyal customer. These are first-party modeled data, as they are derived from modeling observed data that become extremely important smart labels.
Models used in business to extract information from observed data fall into two broad categories: prediction models and detection models.
- Prediction models: provide unpublished information that is not explicitly present in the data. Starting from historical data or from observing the behavior of other customers, these models predict the future behavior of a specific user. For example, they can answer questions about whether or not user X is likely to schedule a call for a product demo or how much user Y will spend in the next few months. The most commonly used prediction models in business are classification models (which classify users into certain categories: will buy the service/won’t buy the service) and regression models (which predict specific future values).
- Detection models: extract features inherent in the data that would not be detectable with the naked eye. They can determine, for example, the type of customer (new, top, at risk of churn) or identify a user’s current interest. To determine customer type, clustering is used, which creates groups of similar users. Common algorithms for clustering include K-means, Hierarchical Clustering, and DBscan. K-means is the simplest and most widely used.
Detection models are also used to detect anomalies; examples include an abnormal increase in sessions or a spike in organic traffic to the website, identified by analyzing time series. To answer complex questions about customer data and optimize marketing and sales strategies, it is essential to choose the right algorithm based on the quantity, quality, and type of data available. The best approach may vary depending on the specific business needs and application context.
Prediction and Detection Algorithms for Business.
The analysis methods that demonstrate the greatest effectiveness and impact in the business context are: classification, regression, clustering, and a combination of NLP and clustering.
Classification
Classification models estimate the probability that an individual belongs to a particular class. They are particularly useful for making decisions based on predictive data. For example, to determine whether a user will subscribe to a newsletter, the algorithm estimates the probability of subscription based on variables such as browsing behavior, type of device used, browser, size of company in which he or she works, and last site visit. If the probability is high, the algorithm classifies the user as a likely enrollee. In addition, the algorithm identifies the most influential variables in the model, providing essential information for optimizing marketing strategies.
Regression
This analysis makes it possible to estimate the value of a variable for an individual based on historical data. For example, by analyzing a user’s past behavior and comparing it with similar users, it is possible to predict how many products they will buy in the next six months. Again, these are predictive algorithms.
Clustering
Clustering is an unsupervised machine learning technique that groups individuals into clusters, so that those within a group are as similar as possible to each other and as different as possible from other groups. This division is useful for targeted marketing actions. Clustering algorithms do not make predictions but highlight intrinsic characteristics in individuals, allowing them to identify, for example, inexpensive or premium customers based on their characteristics, behavior, interests, and past transactions.
NLP+Clustering
By combining Natural Language Processing (NLP) algorithms and clustering, one or more interests can be assigned to each customer or user. For example, by analyzing page views on a site with thousands of thematic pages, one can avoid getting an endless list of interests by clustering pages into macro categories. At Bytek, this process starts with an NLP algorithm that analyzes and understands the content of pages, followed by a clustering algorithm that groups pages by topic, thus facilitating the identification of user interests for marketing actions.
Implementation of prediction and detection algorithms in business
The algorithms analyzed form the fundamental pillars for developing effective strategies not only for marketing and sales, but for the entire business. Specific examples of the application of these algorithms in concrete business contexts are presented in the paragraphs below.
Analysis of abandonment points in onboarding processes.
By using first-party data to feed scoring and churn algorithms, a financial or insurance institution with a fully digital process can identify trouble spots in onboarding processes. This analysis enables targeted engagement and recovery strategies to be activated, significantly improving conversion rates in customer acquisition processes.
Scoring helps determine a user’s likelihood of conversion, while the churn algorithm predicts whether the user is about to leave the onboarding path. This is particularly useful for financial or insurance institutions that operate through digital onboarding funnels. A user who enters the onboarding process and receives a high propensity score, based on his or her behavior and that of other converted users, is considered a valuable potential customer with a high likelihood of activating a current account or seeking advice.
If the churn rate increases during the user’s browsing path, this indicates likely user dissatisfaction, which could be due to difficulties in finding documents or other issues. In this scenario, artificial intelligence algorithms signal that the customer is too important to be lost, allowing retargeting strategies, email marketing, customer experience personalization, mobile marketing and other tactics to be activated to reduce the churn rate and bring the user back into the conversion funnel.
Customer churn risk alert
Another significant case study involves the implementation of a churn algorithm focused on existing customers by a brand operating in the retail sector with both a physical and digital presence.
Through the use of artificial intelligence technologies, the company analyzes and interprets shoppers’ behavioral data, categorizing them into specific clusters. This approach makes it possible to develop and execute targeted loyalty strategies, tailored to the profile and needs of each cluster, with the goal of effectively preventing and combating churn.
For the retail chain, it is essential to retain Top Clients, that is, those who make frequent purchases, have been loyal for many years, and have never stopped visiting physical and digital stores. In the event that such a customer stops frequenting the stores for an extended period, the churn rate increases and the company receives an anomaly notification. At this stage, the brand can intervene by sending personalized emails and triggering direct sales actions. The ability to perform these operations on a large scale for thousands of customers makes the investment in artificial intelligence highly scalable and cost-effective.
Prompt Engineering with First Party Data
In the world of Generative AI, models statistically calculate the probability that a word is akin to a previous word. A marketing agency that aims to maximize the effectiveness of Generative AI must create user experiences that accurately reflect the client brand’s values, goals, and audience. This strategic approach allows for the development of innovative and personalized solutions, ensuring that each campaign is perfectly aligned with the brand’s identity and authentically resonates with its customer base, making the most of the potential offered by technology.
To achieve this, it is essential to develop prompts that integrate the values of the client company, the values of the target users, their interests, the cluster for which the message/image is intended, the company’s tone of voice or that of the target audience. This process allows you to create content that can effectively engage the target audience, as it will be perfectly aligned with their expectations and values.
In this way, you truly leverage Generative AI by instructing it with information drawn from models that have extracted the most from the available first-party data, such as target clusters, tone of voice, values and interests.
Omnichannel Personalization
In FMCG (Fast-Moving Consumer Goods), an industry leader aims to create highly personalized content for each communication channel in which it invests. This strategy ensures that the promotional message is optimized to effectively reach and engage the target audience, improving the effectiveness of the communication and maximizing the return on investment on each platform used.
The main goal is to implement effective omnichannel personalization, made possible through the use of first-party data and generative artificial intelligence. For example, by analyzing historical channel performance, if YouTube proves to be performing well with short videos for the brand, this information is used to create specific video prompts. Similarly, if posts with images perform better on Facebook, format information and AI-generated data, such as top customers and their thematic and product interests, are integrated into the prompt. This process enables the production of content tailored to the target audience, brand and channel of choice, ensuring maximum campaign impact.
In the absence of first-party data or effective models, the use of generative AI risks producing generic content with poor performance and little adherence to business needs.
In addition, it is critical to choose software solutions and platforms that are connected to the external ecosystem, such as ADV platforms, CRMs, and corporate websites. Without these connections, you risk effective models that remain confined to dashboards, offering interesting but not really actionable analytics.
The Bytek Prediction Platform aims to solve this problem by combining centralized and integrated first-party data with proprietary prediction and detection algorithms. The informations obtained are transformed into actionable intelligent attributes on various marketing platforms thanks to connection modules, thus ensuring maximum campaigns effectiveness.
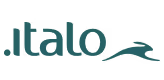